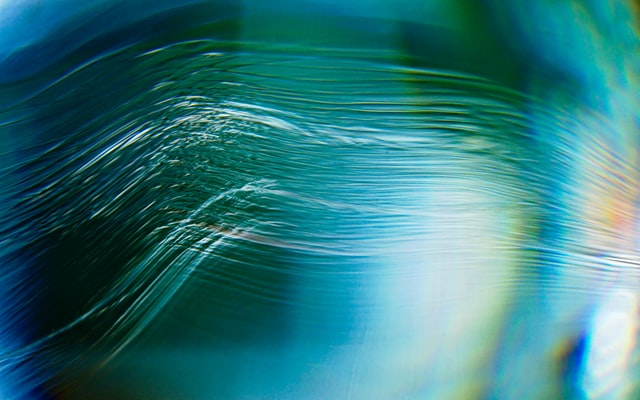
You would have to be hiding under a rock these days not to notice all of the hype around artificial intelligence.
I am not trying to belittle this important technology, but I am sounding the alarm about the oversimplification of this technology innovation.
Conventional wisdom suggests that applying machine learning models and AI to address a business issue without human intervention is a total game-changer. Technology vendors have been promoting fully autonomous systems that can supplant the work of experienced personnel.
The truth is more complicated. In reality, there are multiple ways organizations will be able to use powerful techniques and models to transform the untapped value of data.
There are, of course, many situations when we can use machine learning models to automate clear and predictable business processes. For example, when browsing an online store, we have all received suggestions for similar items to consider – typically, these items appear because of a machine learning algorithm. However, many more significant processes require human judgment to improve decision-making because humans can bring context to complex problems, make connections from similar situations they’ve encountered, and offer recommendations.
Our team felt compelled to write a book about what we call Augmented Intelligence to clarify what is possible with artificial intelligence and what is not. Our book’s thesis is that the true power of artificial intelligence and machine learning lies in humans’ ability to work collaboratively with advanced technologies to create the best possible outcomes.
Things are always more complicated than we would hope, especially when it comes to the promises of new technologies. After more than 30 years as a consultant and analyst in emerging technologies, I’ve learned that the implementation of promises is always more difficult and problematic than we expect.
How is this playing out with machine learning models? First, there is the basic problem of the data itself. Without a clear cycle of data management, machine-learning models may cause more problems than they solve. You must then think about how a business can explain how decisions derived from a machine learning model were made.
Here are a few questions that may arise:
- – What are the hidden and not so hidden biases with the data selected to create a model?
- – What are the ethical challenges that must be managed as we move to this brave new world of Artificial Intelligence?
- – For example, how can a manager explain what business processes and rules are behind a model? Are those rules ethical?
- – Do these models adhere to either corporate or governmental requirements?
- – How does an organization know if there is bias in a model that can impact business outcomes?
It is not enough to simply proclaim that an organization selected just the right data. One person’s determination that the data is correct can be very different from another person’s assumptions.
Two categories of augmented intelligence
We classify augmented intelligence into two categories – weak augmentation and strong augmentation.
Weak augmentation:
Straight forward business processes that are predictable enough to use and rely on automated AI are considered weak augmentation. This might be a model that determines when a customer should be routed to a live agent for support. The model learns about the types of questions that are easily understood and those that require intervention.
Strong augmentation:
On the other hand, a process is classified as strong augmentation when the problem and subject matter are so complicated that humans must collaborate with the AI-based system. For example, an AI model can read a chest x-ray with great precision, but a human might spot a different condition, like a lump that required further exploration. The physician who has been treating a patient will be in the best position to look at the AI-assisted system results while also reviewing the x-ray and relying on their own experience.
It is true that AI models are transforming the way we can use data to solve a huge variety of problems based on automating processes. However, the most successful businesses will use a combination of model-based automation combined with augmented intelligence to create innovation.